The Statistical Basis of Compassion
- talbot0
- Jun 10, 2016
- 2 min read
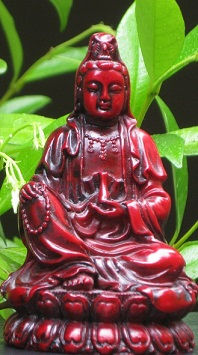
In the March 2016 issue of Educational Researcher, Gene Glass summarizes decades of meta-analyses on a variety of educational approaches. Meta-analyses compare a large number of studies on the same topic, and compute the average benefit one approach has over another. His core finding: The variation in effect sizes for a given intervention tends to be greater than the effect size itself.
His example is bilingual education. Developmental bilingual ed has a mean positive effect over single-language learning of .18 standard deviation. (Proportions of the standard deviation—essentially the average amount scores vary from the mean—is a common way to compare studies that use different outcome measures.)
However, the standard deviation of the 30 meta-analyzed studies (that’s right-- the standard deviation of 30 standard-deviation effect sizes) is .86, almost five times the size of the mean. That means if a school system decided to institute bilingual education with nothing else to go on besides the average effect size, benefits might be anywhere from very positive (.18+.86=1.04 standard deviation) to very negative (.18-.86=-.68). Glass says this is not an atypical finding, and his conclusion is that we should accept that quantitative theory can only do so much, education being necessarily a mix of art and science.
I had two reactions to the article. One was that the pooled results remind me of findings within individual studies. It’s not unusual for attitudes or scores, particularly around new ideas or concepts, to vary more than they converge. Distributions can be so skewed or lumpy that the mean does not represent any kind of norm, and we have to use other statistics to describe the results. We often assume that studying more people (by recruiting them or by pooling studies) will “smooth out” the curve and reveal the “true” effect or parameter.
Combining my experience with Glass’ observations, my other reaction was that variation in learning has a fractal nature. The large-scale patterns are made up of small-scale patterns with similar appearance. We can’t smooth out the variability, because variability is the parameter. This doesn’t mean we shouldn’t look for average effects. As I argue elsewhere in these posts, policy makers with limited budgets have to go after the greatest good for the greatest number. But with an intervention that can only address a slice of a non-normal distribution, the greatest number may still exclude as many or more people than it serves. So treat your teachers, students, researchers, and program evaluators with compassion. We are all outliers here.
Comments